The X Vector Architecture For Speaker Recognition Using Joint

The X Vector Architecture For Speaker Recognition Using Joint The x vector architecture for speaker recognition. using joint learning, the frame level information from the tdnn layer is compared with the center vector in the ppl layer to calculate the ppl. X vectors: robust neural embeddings for speaker recognition by david snyder a dissertation submitted to the johns hopkins university in conformity with the requirements for the degree of.
The X Vector Architecture For Speaker Recognition Using Joint The x vector system. in comparing with x vectors, we also contribute a study of augmentation in i vector systems. 2. speaker recognition systems this section describes the speaker recognition systems developed for this study, which consist of two i vector baselines and the dnn x vector system. all systems are built using the kaldi speech recog. Following the state of the art approaches reviewed in section 2, we designed speaker embedding based solutions, which are further selected for experimental evaluation on the text independent sv task. standard d vector, r vector and x vector based systems as well as a variety of their modifications are examined. 2. x vector system 2.1. overview the x vector system is based on a framework that we de veloped for speaker recognition [11]. the system is com prised of a feed forward dnn that maps variable length speech segments to embeddings that we call x vectors. once extracted, the x vectors are classified by the dis. X vector based speaker diarization using bi lstm and interim voting driven post processing authors : j. b. mala , s. m. alex raj , rajeev rajan authors info & claims text, speech, and dialogue: 27th international conference, tsd 2024, brno, czech republic, september 9–13, 2024, proceedings, part ii.
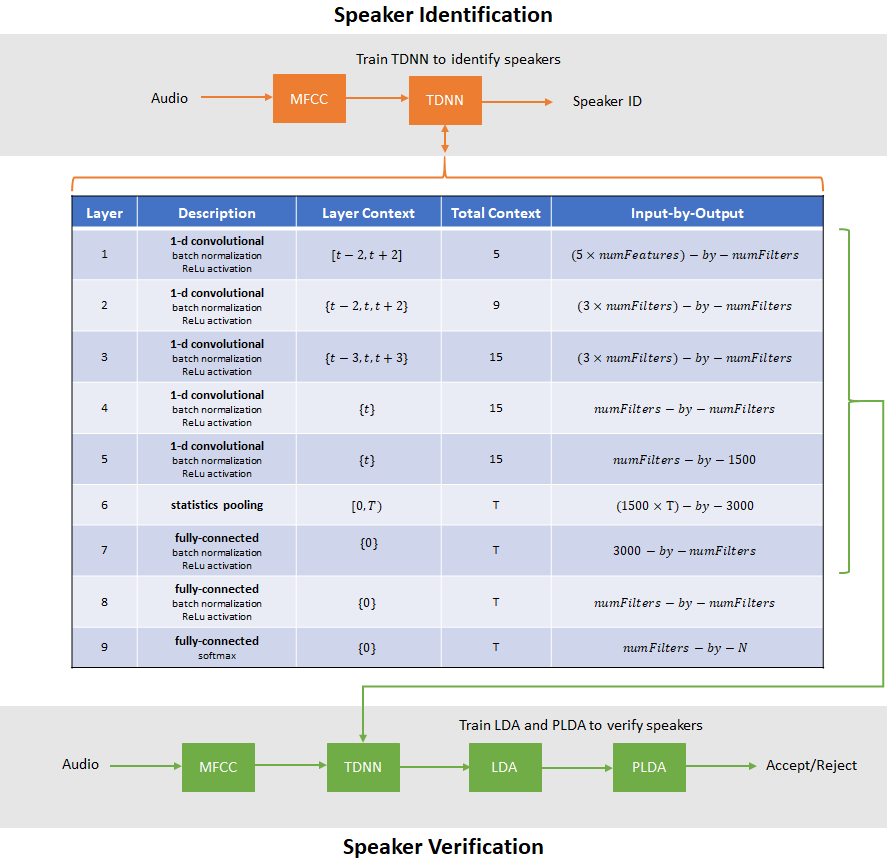
ççspeaker çü ççrecognition çü ççusing çü ççx çü ççvectors çü Matlab Simulink Mathworks õ õø 2. x vector system 2.1. overview the x vector system is based on a framework that we de veloped for speaker recognition [11]. the system is com prised of a feed forward dnn that maps variable length speech segments to embeddings that we call x vectors. once extracted, the x vectors are classified by the dis. X vector based speaker diarization using bi lstm and interim voting driven post processing authors : j. b. mala , s. m. alex raj , rajeev rajan authors info & claims text, speech, and dialogue: 27th international conference, tsd 2024, brno, czech republic, september 9–13, 2024, proceedings, part ii. The new dnn based version of vocalise using x vectors provides a powerful, flexible tool for automatic speaker recognition it maintains an open box philosophy and allows the forensic practitioner to interpret their speaker recognition results in a likelihood ratio framework. significant performance improvements are observed using the new. In this paper, we use data augmentation to improve performance of deep neural network (dnn) embeddings for speaker recognition. the dnn, which is trained to discriminate between speakers, maps variable length utterances to fixed dimensional embeddings that we call x vectors. prior studies have found that embeddings leverage large scale training datasets better than i vectors. however, it can.
Comments are closed.