Table 2 From Deeply Fused Speaker Embeddings For Text Independent
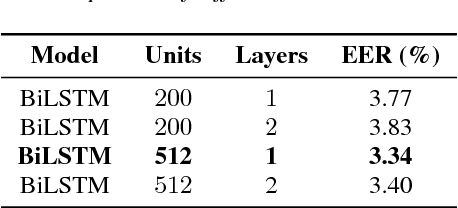
Table 2 From Deeply Fused Speaker Embeddings For Text Independent In this work we propose deeply fused speaker embeddings speaker representations that combine neural speaker embeddings with i vectors. we show that by combining the two speaker representations we are able to learn robust speaker embeddings in a computationally efficient manner. we compare several different fusion strategies and find that the. Deeply fused speaker embeddings for text independent speaker verication. deeply fused speaker embeddings for text independent speaker verication. gautam bhattacharya1 ;2, jahangir alam2, vishwa gupta2, patrick kenny2 1mcgill university. 2computer research institute of montreal (crim) [email protected].
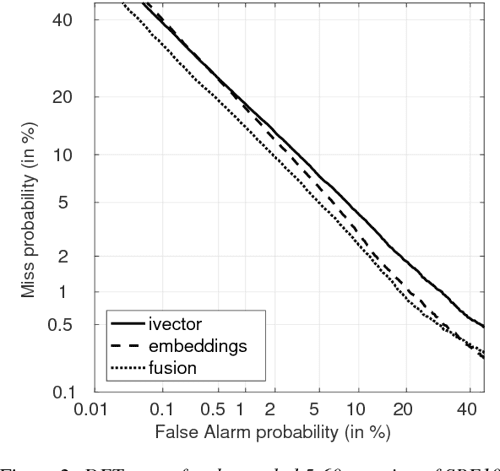
Pdf Deep Neural Network Embeddings For Text Independent Speaker Deeply fused speaker embeddings for text independent speaker verification. september 2018. doi: 10.21437 interspeech.2018 1688. conference: interspeech 2018. authors: gautam bhattacharya. mcgill. This article applies speaker embeddings to the task of text independent speaker verification, a challenging, real world problem in biometric security by combing a novel 1 dimensional, self attentive residual network, an angular margin loss function and adversarial training strategy. expand. 37. [pdf] 2 excerpts. As a state of the art solution for speaker verification problems, deep neural networks have been usefully employed for extracting speaker embeddings which represent speaker informative features. objective functions, as the supervisors for the learning of discriminative embeddings, play a crucial role for this purpose. in this paper, motivated by the success of metric learning approaches, we. Table iii shows the text independent speaker verification performance of opt tdy resnet 34(×0.25) using following temporal pooling methods: average pooling [71][72][73], statistical pooling [1,74.
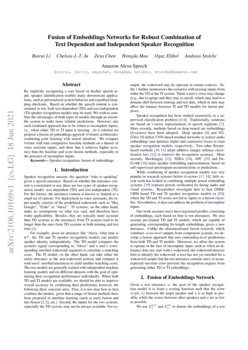
Fusion Of Embeddings Networks For Robust Combination Of Text Dependent As a state of the art solution for speaker verification problems, deep neural networks have been usefully employed for extracting speaker embeddings which represent speaker informative features. objective functions, as the supervisors for the learning of discriminative embeddings, play a crucial role for this purpose. in this paper, motivated by the success of metric learning approaches, we. Table iii shows the text independent speaker verification performance of opt tdy resnet 34(×0.25) using following temporal pooling methods: average pooling [71][72][73], statistical pooling [1,74. Frame level speaker embeddings for text independent speaker recognition and analysis of end to end model suwon shon, hao tang, james glass computer science and articial intelligence laboratory massachusetts institute of technology cambridge, ma 02139 usa fswshon,haotang,glass [email protected] abstract in this paper, we propose a convolutional neural. Deep embedding based text independent speaker verification has demonstrated superior performance to traditional methods in many challenging scenarios. its loss functions can be generally categorized into two classes, i.e., verification and identification. the verification loss functions match the pipeline of speaker verification, but their implementations are difficult. thus, most state of the.
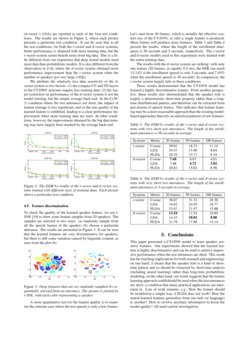
Deep Speaker Feature Learning For Text Independent Speaker Verification Frame level speaker embeddings for text independent speaker recognition and analysis of end to end model suwon shon, hao tang, james glass computer science and articial intelligence laboratory massachusetts institute of technology cambridge, ma 02139 usa fswshon,haotang,glass [email protected] abstract in this paper, we propose a convolutional neural. Deep embedding based text independent speaker verification has demonstrated superior performance to traditional methods in many challenging scenarios. its loss functions can be generally categorized into two classes, i.e., verification and identification. the verification loss functions match the pipeline of speaker verification, but their implementations are difficult. thus, most state of the.
Comments are closed.